Category Archive for: ‘Automobiles’
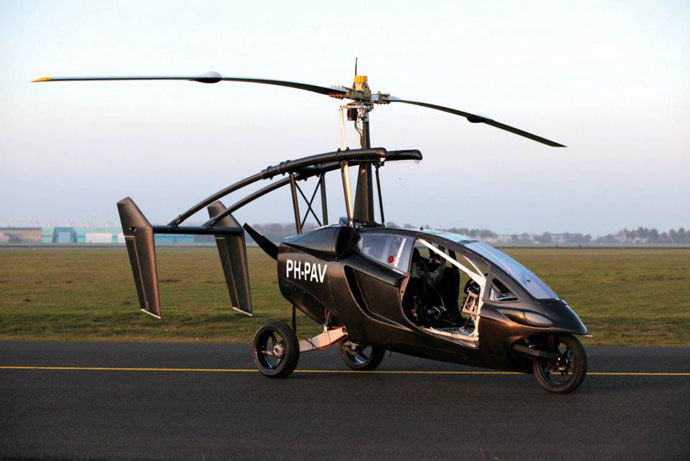
This Badass Hybrid Car And Gyroplane Will Set You Back $295k
Forget about the Batmobile — it's all about the PAL-V One these days. Just like it sounds, this is a three-wheeled car that turns into a helicopter, and you don't have to be a superhero to ……Continue Reading
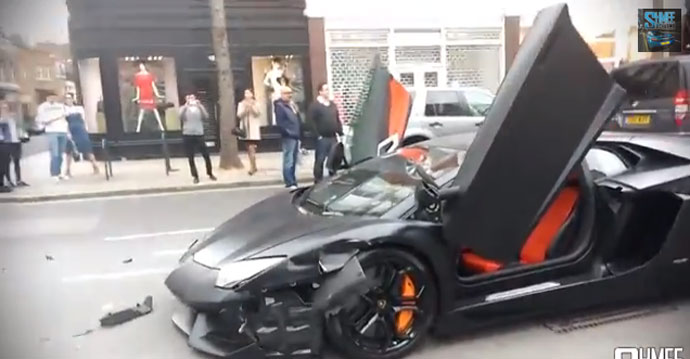
Must-See: London Crash Proves Lamborghinis Can Fly (Video)
Few cars are as liftoff-ready as a $400,000 Lamborghini Aventador. In a crash last week on posh Sloane Street in …
Read More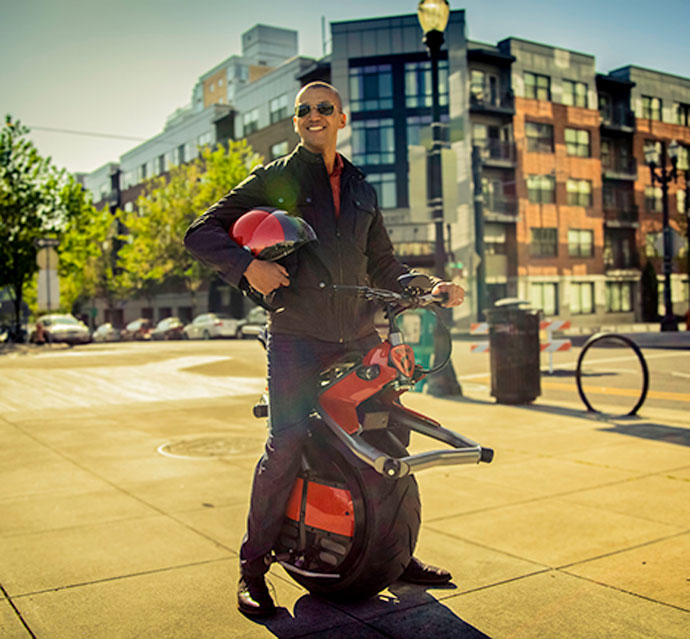
The Ryno Motorcycle Has Just One Wheel
The Ryno one-wheeled motorcycle is the first vehicle of its kind. The electric vehicle has just one wheel, and it …
Read More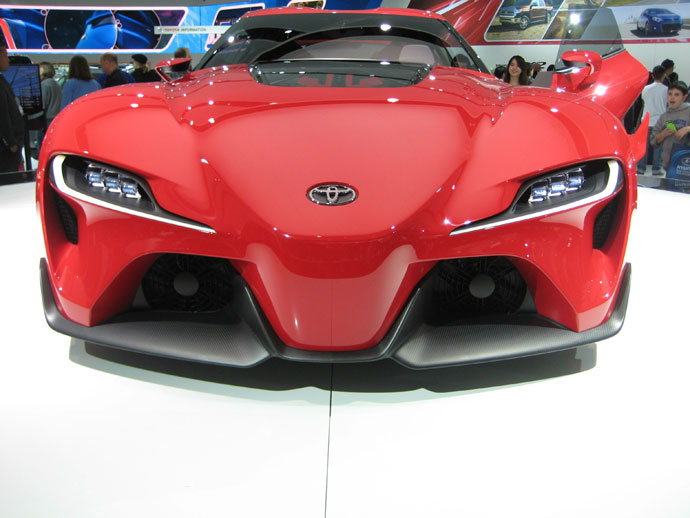
Is This The New Supra? Toyota FT1 Concept Turns Heads In Detroit
During the Detroit Auto Show, Toyota rolled out this FT1 concept car that made lots of noise. Rumor has it …
Read More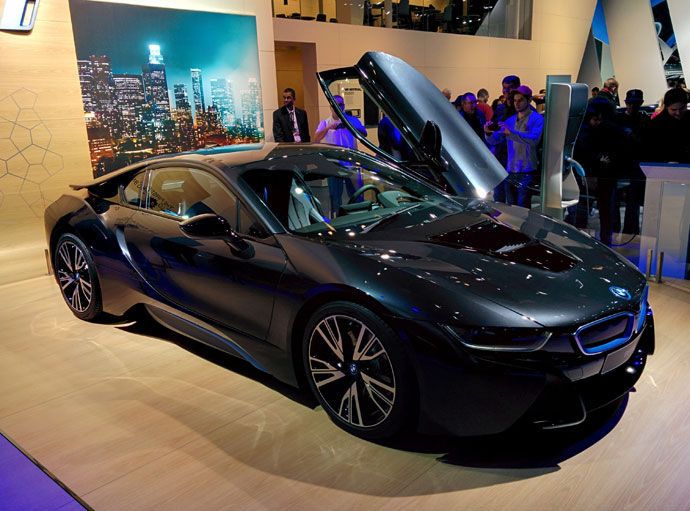
26 Awesome Cars From The 2014 Detroit Auto Show
The North American International Auto Show is in full gear in Detroit right now, showing off the latest and greatest …
Read More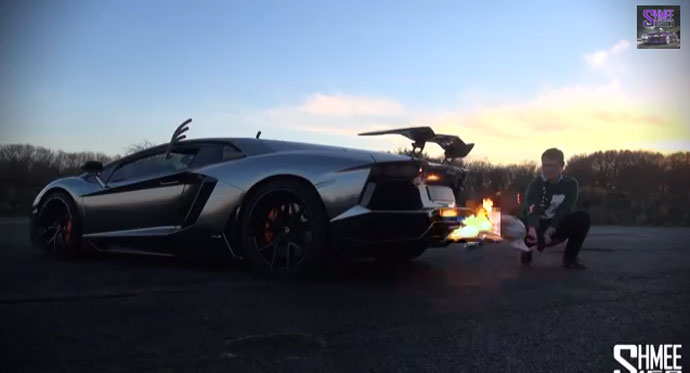
Lamborghini Aventador Flames Roast Turkey (Video)
Car blogger Tim Burton wanted to try something new for Christmas dinner this year. He skewered a turkey with a …
Read More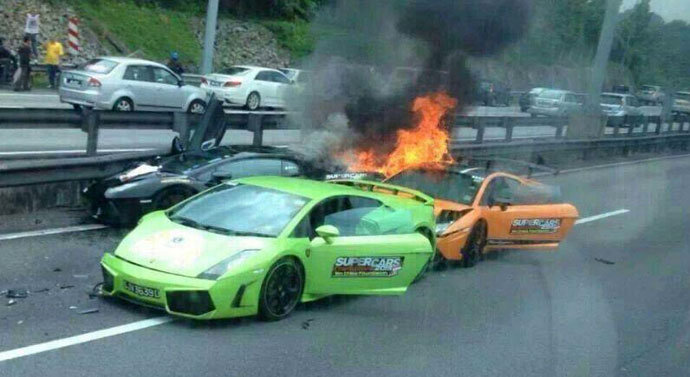
3 Lamborghinis Collide And Burn To A Crisp
This week has been marked by a number of incidents involving high end sports cars. Just a few days ago, …
Read More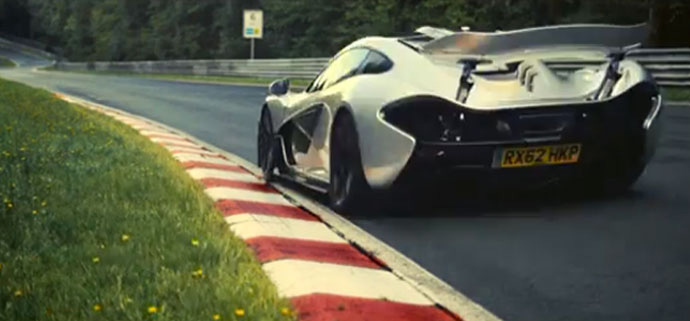
McLaren P1 Supercar Screams Through The World’s Toughest Race Track
McLaren has been known for building amazing vehicles. Its F1 supercar was a much to be desired exotic vehicle, and …
Read More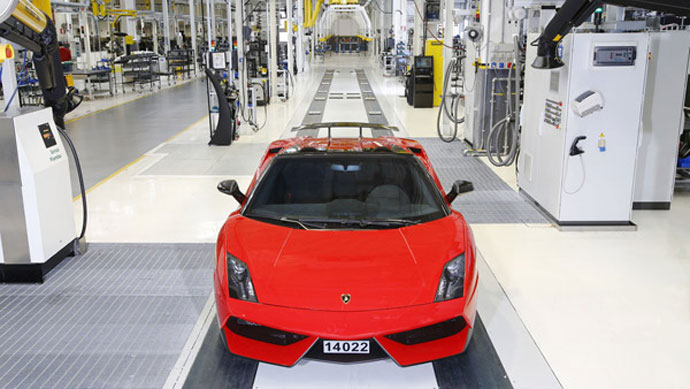
Production of Lamborghini Gallardo Ends
Yesterday marked the end of an era for Lamborghini and car lovers worldwide, as the very last Gallardo rolled off …
Read More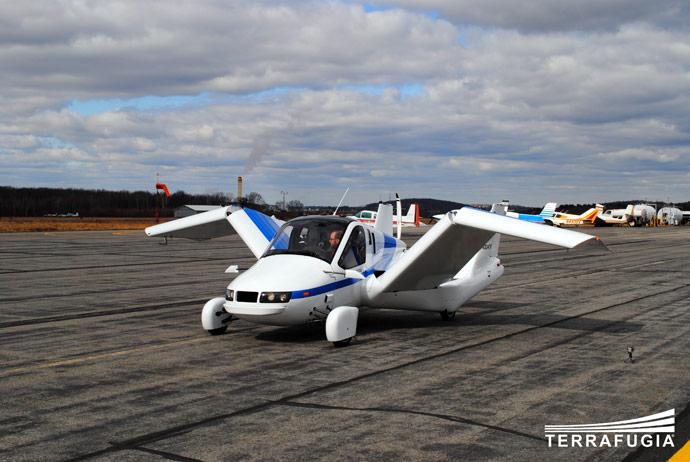
Flying Car Is Now A Reality
Terrafugia, a privately held American company, has been developing something that has been a dream of mankind for many years …
Read More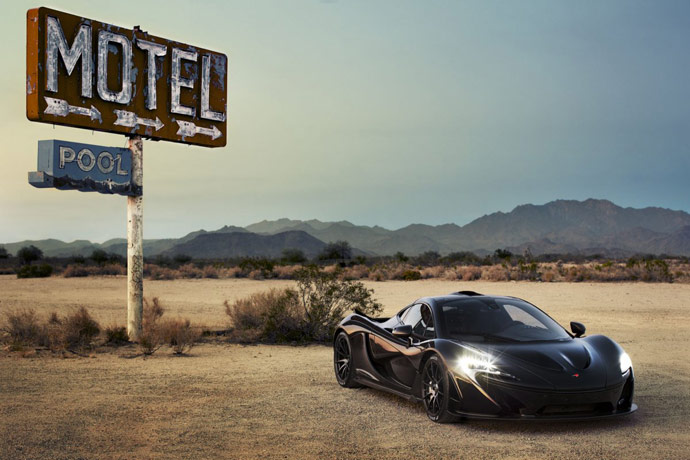
McLaren P1 Supercar Tested In The Desert Heat
McLaren has been working on it’s Supercar, the P1, for just about a year now. And the fact that it’s …
Read More